Title
2021 Stemmler Fund Projects & Recipients
Title
2021 Stemmler Fund Projects & Recipients
Automated Assessment of Written Chart Notes: Generating Reliable, Timely, and Useful Feedback
This project aims to develop, implement and evaluate new ways to extend the capabilities of ASAG systems using advances in natural language processing and machine learning to rapidly and accurately assess learners’ responses in the patient note.
History styling
history-white-theme-paragraph
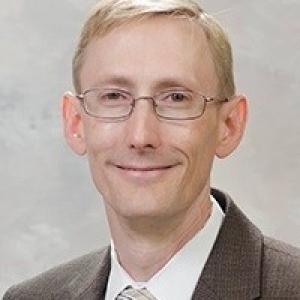
William Bond, MD, MPH
Director of Research, Jump Simulation
University of Illinois of Chicago SOM
University of Illinois of Chicago SOM
History styling
history-white-theme-paragraph
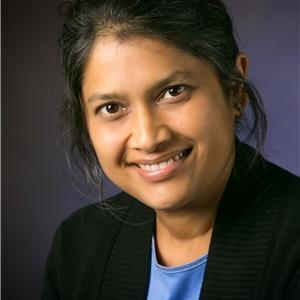
Suma Bhat, PhD
Assistant Professor
University of Illinois at Urbana-Champaign
University of Illinois at Urbana-Champaign
History styling
history-white-theme-paragraph
Download this project's abstract by clicking the link below.
Exploring Validity Evidence for the use of Immersive Virtual Environments for Formative and Summative Assessment Purposes
This project will explore the validity argument and formative value of immersive virtual environment while also demonstrating a process others might use in their own contexts.
History styling
history-white-theme-paragraph

Walter Tavares, PhD
Assessment Scientist and Assistant Professor
University of Toronto
University of Toronto
History styling
history-white-theme-paragraph

Fahad Alam, MD
Medical and Research Director
Sunnybrook Canadian Simulation Centre
Sunnybrook Canadian Simulation Centre
History styling
history-white-theme-paragraph
Download this project's abstract by clicking the link below.
Bias Reduction in Curricular Content: Using Machine Learning and Artificial Intelligence to Assess Bias in Medical Education
This project will explore the use of machine learning and artificial intelligence as ways to reduce bias in medical education.
History styling
history-white-theme-paragraph
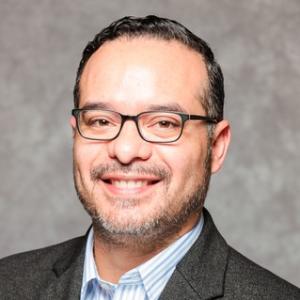
Roberto Montenegro, MD, PhD
Assistant Professor, Child and Adolescent Psychiatry; Associate Director, Bias Reduction in Curricular Content (BRICC)
Seattle Children’s Hospital Foundation
Seattle Children’s Hospital Foundation
History styling
history-white-theme-paragraph